Introduction
To solve any machine learning problem we need three ingredients: some data observed in the world, a model to explain our data, and an optimizer that can fit the model to the data. In this blog we re-visit the optimization problem, which we first explained in the Gradient Descent post. We present the problem of constrained optimization and explain the conditions required to achieve an optimal solution when solving constrained optimization problems.
Humans approximately solve constrained optimization problems frequently. For example, if someone wants to find a way to get to the city as quickly as possible without paying highway tolls, that person would be solving a constrained optimization problem. Specifically, the objective is to get to the city as quickly as possible, and the constraint is to not use any roads or highways that might require the person to pay tolls. Such problems are commonplace and require a search over all solutions to verify that the solution is optimal and does not violate any of the constraints. From a computational perspective these constrained optimization problems are NP-hard.
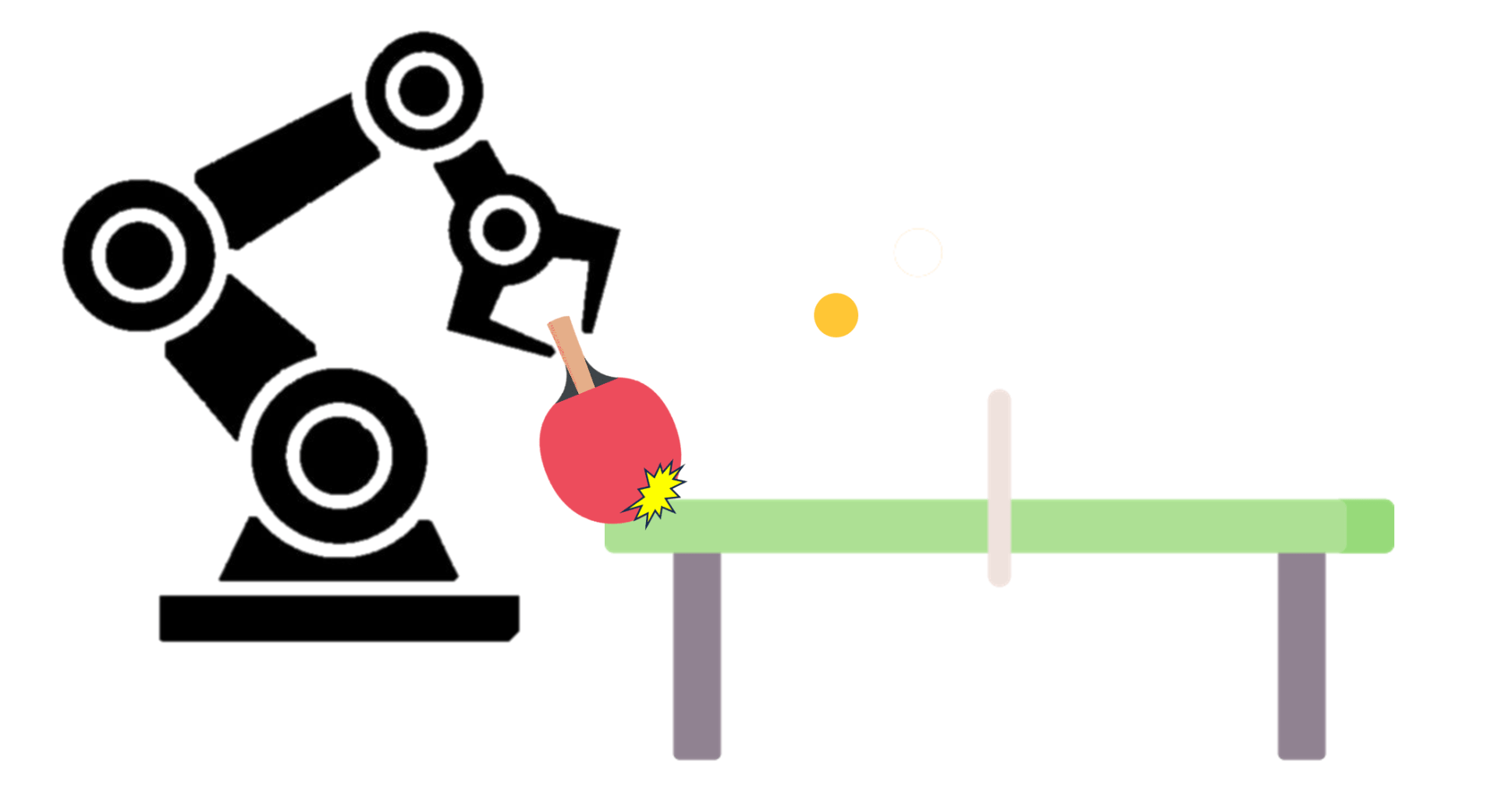
These problems are also present in robot learning. Consider a robot learning to hit a table-tennis ball from demonstrations (Figure 1). We can collect demonstration data of a human moving the robot’s arm through the motion of a table tennis stroke. We might then have a supervised learning model to learn the task by minimizing the distance between the demonstrated and learned robot trajectories. However, there are also several possible constraints to this learning problem. First is a constraint of physics, such as the robot’s forward dynamics model. The forward dynamics model for a robot specifies how much its joints can move under a given force. These can be thought of as equality constraints, $\tau = H(q)\ddot{q}$, where a given torque, $\tau$, specifies the amount a robot joint can move via a joint-space inertia matrix, $H$, whose variables are the robot’s joint angles $q$. Other specific constraints might be that the robot needs to learn these trajectories safely, for example, the robot should never hit the table while playing (Figure 1). This could be done by creating a box around the table and telling the robot to never enter the box. Such a constraint might be an inequality constraint, i.e., $x < 10$, where $x$ is the robot's end-effector position along the $x$-coordinate and at $10m$ might be the edge of the table w.r.t. to the origin of the robot's coordinate frame. Hence the learning problem can have both equality and inequality constraints. Such an optimization problem can be specified as: \begin{alignat*}{3} & \text{minimize}& f(\mathbf{x})\\ & \text{subject to} \quad& h_j(\mathbf{x}) = 0, \forall j\\ & \quad& l_i(\mathbf{x}) \leq 0, \forall i, \label{eq:constrained_optimization} \end{alignat*} where the constraints for the robot's dynamics ($h_j$) and physical space ($l_i$) are specified and the goal would be minimize a loss function for the supervised scenario where the robot generates a table-tennis trajectory similar to the human's demonstration.
Equality Constraints in a learning problem

We first examine the solution to the constrained optimization problem considering only equality constraints. Consider the problem of form:
\begin{alignat}{3}
& \text{minimize} \quad f(x_1,x_2) \nonumber \\
& \text{subject to} \quad h_0(x_1,x_2) = c \quad .
\end{alignat}
In this example vector $\mathbf{x} = [x_1,x_2]$, and there is only one constraint $h_0$. Figure 2 provides an example function $f(\mathbf{x})$, and its equality constraint $h(\mathbf{x})$. Notice that the optimal point for the function under the constraint is a point where the constraint function is tangent to the isoline with value $d_1$. This is because moving along the equality constraint does not make the values of the function $f(\mathbf{x})$ increase in either direction, meaning that this is a stationary point. An optimal value of the function under constraints can only exist on such stationary points. This stationarity condition is a necessary condition for optimality. Further, the gradient of the function is parallel to the gradient of the equality constraint, as the two curves, i.e, the optimization function and constraint, are tangent at these stationary points. If the two curves are tangent, their gradients vectors differ only by a scalar, i.e., $\nabla f = \lambda \nabla h$. This property is shown in Figure 2 more clearly.
The Lagrangian function can then be defined as:
\begin{equation}
\mathcal{L}(\mathbf{x},\lambda) = f(\mathbf{x}) + \lambda h(\mathbf{x}),
\end{equation}
where the necessary, but not sufficient, condition for optimality is $\nabla_{\mathbf{x}, \lambda} \mathcal{L}(\mathbf{x}, \lambda) = 0$. We can now use gradient descent to find a locally optimal solution for the Lagrangian function, which will provide us with solution for both, the parameter $\lambda$, and values for the vector $\mathbf{x}$ for the locally optimal solution. If the two functions, $f$ and $h$ are convex, then the solution will be a globally optimal solution. This technique of converting a constrained optimization problem into the new function where the constraints are summed is called the Lagrange multipliers technique. The Lagrange multipliers technique hence allows us to use gradient descent to solve a constrained optimization problem, which allows for a computationally feasible way to solve these problems, without the use of matrix inversions.
Inequality constraints in the optimization problem
To solve constrained optimization problems with inequality constraints, we again use Lagrangian multipliers. Consider the following constrained optimization problem:
\begin{alignat}{3}
& \text{minimize}& f(\mathbf{x}) \nonumber \\
& \text{subject to} \quad& h_j(\mathbf{x}) = 0, \forall j \nonumber \\
& \quad& l_i(\mathbf{x}) \leq 0, \forall i
\end{alignat}
This is the primal problem, where we are minimizing the function w.r.t. to the input variable $\mathbf{x}$. However, a dual problem can be formulated, which is maximized w.r.t. to the constraint variables. Sometimes the dual problem might make the primal problem computationally tractable, or vice versa, because the evaluation in one of the forms for the function being computed might be easier.
A dual problem changes the variables of the optimization problem and the direction of the optimization from a minimization problem to a maximization problem. The value of the primal and the dual problem at the optimal solution are equal, where the minimization problem provides the upper bound, and the maximization problem, i.e., the dual, presents the lower bound for the values of the primal problem.
We formulate the dual with the Lagrangian:
\begin{equation}
\mathcal{L}(\mathbf{x},\mathbf{\lambda},\mathbf{\alpha}) = f(\mathbf{x}) + \sum_j \lambda_j h_j(\mathbf{x}), + \sum_i \alpha_j l_i(\mathbf{x}).
\end{equation}
The dual function using this Lagrangian would be:
\begin{equation}
g(\mathbf{\lambda},\mathbf{\alpha}) = \textrm{min}_x \mathcal{L}(\mathbf{x},\mathbf{\lambda},\mathbf{\alpha}),
\end{equation}
and the dual optimization problem would be:
\begin{alignat}{3}
\lambda^*\!, \alpha^* =& \text{argmax}_{\mathbf{\lambda}, \mathbf{\alpha}} g(\mathbf{\lambda}, \mathbf{\alpha}) \nonumber \\
& \alpha_i \geq 0, \forall i .
\label{eq:dual_prob}
\end{alignat}
To find the optimal solution for the constrained optimization problem we need to find values of $\mathbf{x},\mathbf{\lambda},\mathbf{\alpha}$ at which the Lagrangian is stationary, and both the primal and dual problems are satisfied without any constraint violations. We use gradient descent iteratively to solve this problem:
[Step 1] Find the optimal value of $\mathbf{x}$ under which the Lagrangian is minimized, i.e, $\mathbf{x}* = \textrm{argmin}_{\mathbf{x}} \mathcal{L}(\mathbf{x},\mathbf{\lambda},\mathbf{\alpha})$. We pick the values for $\mathbf{\lambda}$ and $\mathbf{\alpha}$ at random for the first iteration.
[Step 2] Holding the optimal value of $\mathbf{x}*$ found previously, we now find the gradient of $\mathcal{L}$ w.r.t. $\mathbf{\lambda}$ and $\mathbf{\alpha}$, and update their values with a learning rate to perform gradient ascent. This is akin to moving slowly towards the optimal solution of the dual problem defined in Eq. \ref{eq:dual_prob}.
We iterate over both these steps until we reach the stopping conditions, which are called the Karush–Kuhn–Tucker (KKT) conditions for optimality. These conditions are:
- Stationarity: The gradient of the Lagrangian is zero as explained in the previous section, i.e., $\nabla \mathcal{L} = 0$.
- Primal feasiblity: The constraints of the primal problem are satisfied, i.e., $h_j(\mathbf{x}) = 0, \forall j,$ and $l_i(\mathbf{x}) \leq 0, \forall i$.
- Dual feasiblity: The constraints of the dual problem are satisfied, i.e., $\alpha_i \geq 0, \forall i$.
- Complimentary Slackness: $\sum_i \alpha_j l_i(\mathbf{x}) = 0$. This ensures that either the inequality constraints in the primal are satisfied by equality, i.e., are tight, and $\alpha_i$ is unbounded, or $\alpha_i = 0$, and the constraint has a lot more slack in satisfiability.
The KKT conditions provide the necessary conditions for local optimality for a constrained optimization problem with inequality constraints. These conditions were developed as a generalization to the Lagrange Multipliers technique that account for both equality and inequality constraints. The Lagrange multipliers technique developed in the previous section is a technique only applicable to equality constraints.
We can compute the solution of the primal and the dual at each iteration of the optimization problem, and also compute the optimality gap: $\frac{p^k-d^k}{d^k}$, where $p^k$ and $d^k$ are the values of the primal and dual problems in iteration $k$ of the optimization. Here the solution of the minimization primal problem provides the upper bound of the function value and the solution of the dual provides the lower bound of the function value, which converge towards each other asymptotically over optimization iterations. The optimality gap provides a range within which the optimal constrained solution lies and gives us an upper and lower bound over our solution quality.
Key Takeaways
- Constrained optimization is an important and computationally challenging problem needed for robot and machine learning.
- Given a constrained optimization problem, a Lagrangian can be formulated where the constraints are used as additive factors to the optimization function. The stationary points of the Lagrangian are candidates for the optimal solution of the optimization problem.
- The Lagrange multipliers technique allows us to use gradient descent to solve a constrained optimization problem, which allows for a computationally feasible way to solve these problems by avoiding matrix inversions. These solution methods take a different form to solve approximately for non-convex problems which are generally faster.
- Karush–Kuhn–Tucker (KKT) conditions provide the necessary conditions for local optimality for a constrained optimization problem with inequality constraints. These conditions were developed as a generalization to the Lagrange Multipliers technique.